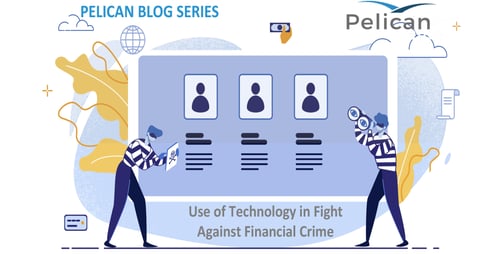
Over recent years, technologies, such as Pelican’s AI platform, which uses Natural Language Processing and Machine Learning, have matured and evolved to a point where they can now mimic how a human can think and decision whilst concurrently addressing key concerns.
“We must make better use of technology, to make us more effective and efficient in weeding out criminal activity. Big data analytics and machine learning, for example, are reducing false positives that require manual review. This contributes to enhanced productivity and the standardisation of compliance efforts.”
David Lewis, Executive Secretary, FATF
January 18, 2021
We couldn’t have said it better.
David and FATF have recognised that financial institutions today are reliant on outdated technology in their fight against financial crime.
Yesterday’s Solution
The sheer volume of false positive alerts created by rule-based screening tools has resulted in the need to suppress or risk weight these alerts. However, this increases the potential that real issues are being missed, which is material because it can have tremendous downstream consequences; the most impactful of these, of course, is a bank unintentionally facilitating money laundering and consequently incurring enforcements and reputational damage.
So how have FATF gotten comfortable with financial institutions now relying on new technologies, such as AI, to investigate and decision these alerts?
To answer that question, it is important to consider what’s changed in the last few years. The short answer is that criminals have become more experienced, sophisticated, and technically advanced, driving compliance alert volumes to increase dramatically. To cope with this, institutions have had to add additional headcount to investigate and resolve these alerts. The result is a substantial increase in the cost of compliance.
Today’s Solutions
Over recent years, technologies, such as Pelican’s AI platform, which uses Natural Language Processing and Machine Learning, have matured and evolved to a point where they can now mimic how a human can think and decision whilst concurrently addressing key concerns such as:- The technology works the way it’s supposed to;
- It’s as good as a person or better;
- The banks have control over it (i.e. no unsupervised, unapproved learnings);
- It’s auditable
In summary, technology has come a long way fast and is so much better, more reliable, and stable than it used to be. It’s also now capable of performing at high speed with large throughput.
Furthermore, today’s solutions also address the regulator’s concerns, in that they’re transparent, demonstrable, and explainable. In short, they’re auditable, like everything else in the bank. These concepts are all relatively self-evident but tied. Let’s dig into what each of these mean in this context.
Transparency
Transparency relates to two things- the transparency of the decision-making models or artificial intelligence, and the transparency of the actions made within the model by the compliance team.
Artificial intelligence is understood much better today than it was five years ago. Internal Model Review teams expect to be able to get under the hood of any technology they are considering deploying.
Transparency also covers how the model learns. Unsupervised, unapproved learnings don’t really have a place in investigating a potential breach. All learnings need to be presented clearly, and be approved, prior to being imbedded in your decision making.
The transparency of the compliance teams is another matter. These days, a full audit trail of every action taken within a regulatory technology isn’t just expected, it’s assumed. This includes capturing changes made to decision trees, decisions made by analysts of all levels, escalations, and changes in administrators..
Demonstrability
Tied closely to transparency is demonstrability. It’s now essential to have a thorough and complete audit trail with analytical dashboards, providing the ability to drill down to every action and decision that has been made during the transaction lifecycle removing any question about “who said what, when.".
Explainability
Explainability takes “who, what, when” and elevates it to “why and how” through highly configurable escalations and case management, tied into alert investigation and resolution.
Looking back in the past and just seeing that an alert has been raised and then released by a user of the AI isn’t good enough. Full information is now required on the data, rationale and models that were used.
Five years ago, technology like this didn’t exist. Since then, though criminals evolved, regulatory requirements and technology have also evolved concurrently to better combat this issue.
In his speech, David Lewis cited a case of Covid aid money that was sent by a foreign country to its citizens stranded in Tunisia. The funds, sent in six installments, instead disappeared into a shell company. The financial institution’s staff were overwhelmed with Covid-related alerts and missed the nefarious nature of these transactions.
With an AI technology solution performing an initial analysis of alerts, the majority of the Tunisian bank’s payment alerts could have been investigated and reduced, freeing up their compliance analyst’s time to investigate this nefarious activity.
With the increasing burden of alerts, and the maturity of technology, the risky position now is to not utilise modern technology and to instead rely on people to sift through vast amounts of information in a time-sensitive way. Surely, as David states, it is incumbent on all of us to be more effective and efficient in weeding out criminal activity
.
http://www.fatf-gafi.org/publications/fatfgeneral/documents/mena-reg-tech-2021.html
Amber Sutherland
Business Development & Sales – Europe
Pelican AI